Understanding the Role of Labelling Tools in Machine Learning
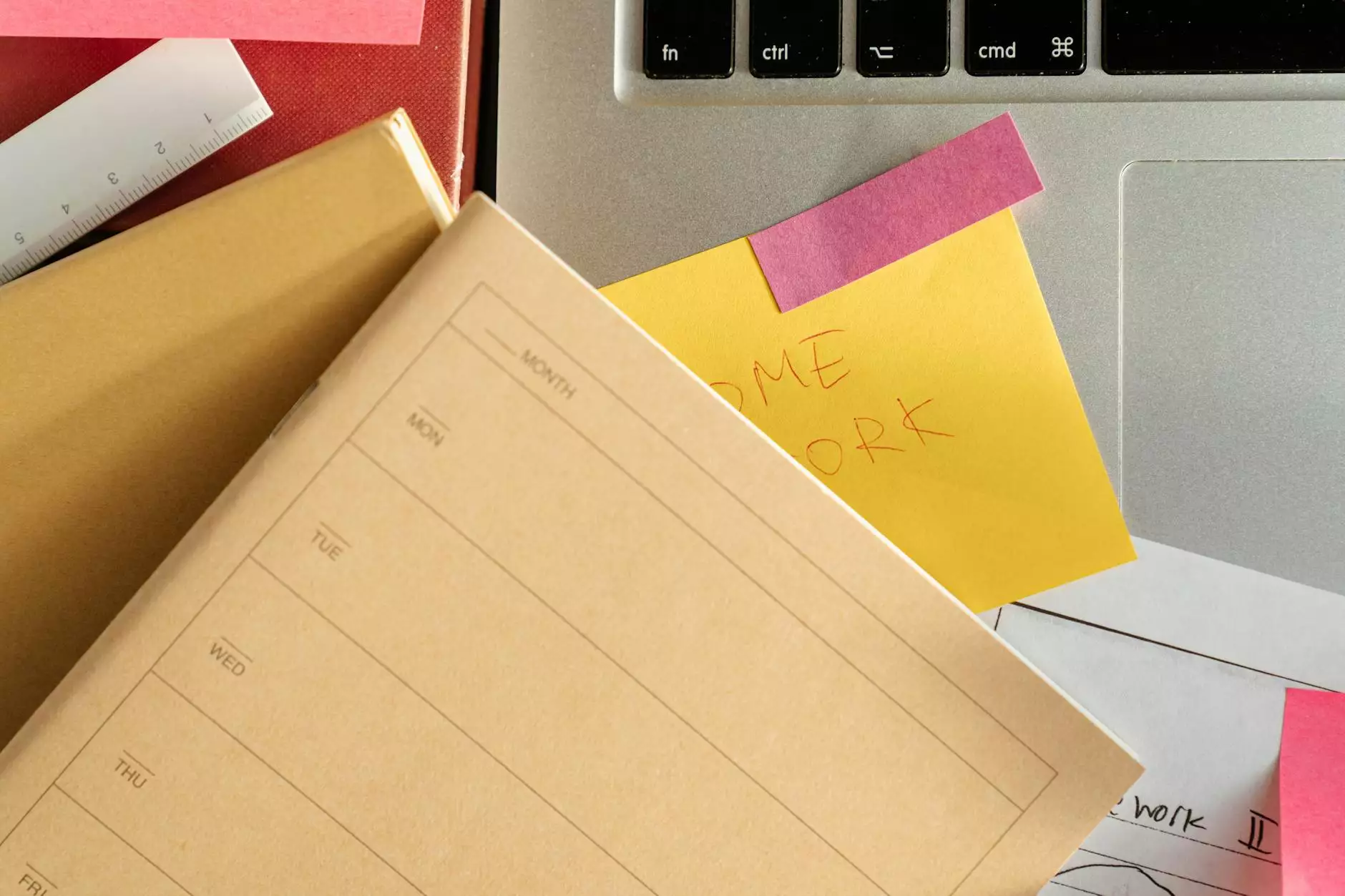
In the realm of machine learning, the importance of data preparation cannot be overstated. Among the numerous steps involved in this preliminary phase, the process of data labelling stands out as crucial for developing reliable machine learning models. In this article, we will delve deep into the concept of labelling tool machine learning, exploring its significance, various tools available, and how Keylabs.ai enhances your data annotation needs.
What is Data Labelling?
Data labelling refers to the process of assigning meaningful tags or annotations to raw data, such as images, text, and audio files. This critical step helps machine learning algorithms understand the patterns and features within the data, leading to improved predictive performance. Without quality labels, an algorithm can struggle to learn effectively, resulting in lower accuracy and functionality.
The Importance of Labelling in Machine Learning
- Enhances Data Quality: Properly labelled data is essential for the accuracy of machine learning models. High-quality labels lead to better generalization and performance.
- Facilitates Supervised Learning: In supervised learning paradigms, the model learns from labelled examples, making data labelling pivotal.
- Boosts Model Interpretability: Well-labelled datasets can help data scientists and stakeholders understand the decision-making process of the algorithms.
- Speeds Up Model Training: Accurate and comprehensive labels can expedite the training process, paving the way for quicker deployments.
The Evolution of Labelling Tools in Machine Learning
With the rapid advancement of artificial intelligence (AI) and machine learning, the demand for efficient labelling tools has skyrocketed. Historically, labelling was a labor-intensive task requiring extensive manual effort. However, advanced technologies have emerged to streamline this process, making it more efficient and less prone to human error.
Modern Labelling Tools and Their Features
Today, several labelling tools utilize machine learning algorithms to assist in data annotation. Let's explore some key features offered by these tools, particularly at Keylabs.ai:
- Automated Labelling: Leveraging AI and machine learning, automated labelling tools can significantly reduce the time required for annotation by suggesting labels based on existing patterns.
- User-friendly Interfaces: Many tools offer intuitive interfaces that simplify the labelling process, allowing non-experts to contribute effectively.
- Scalable Solutions: Whether you have hundreds or thousands of data points, scalable labelling solutions can handle large datasets with ease.
- Collaboration Features: Many platforms include collaborative tools that enable teams to work together seamlessly, ensuring efficient project management.
- Customisable Workflows: Users can tailor workflows according to their specific project needs, making the annotation process more efficient.
Keylabs.ai: Redefining Data Annotation
Keylabs.ai stands out as a premier data annotation platform that provides cutting-edge labelling tools specifically designed for enhancing machine learning projects. The platform combines innovative technology with ease of use to deliver unparalleled labelling solutions.
Why Choose Keylabs.ai?
When it comes to selecting a labelling tool, several factors set Keylabs.ai apart from the competition:
- Quality Assurance: Keylabs.ai maintains a rigorous quality control process that ensures the highest standard of labelled data, critical for developing successful machine learning applications.
- Speed and Efficiency: By utilizing advanced algorithms, projects can be completed much faster, saving valuable time and resources.
- Comprehensive Support: The platform provides exceptional customer support, guiding users through the labelling process and addressing any concerns.
- Affordable Pricing: Keylabs.ai offers competitive pricing plans tailored to different budgets, making high-quality labelling accessible to everyone.
- Versatile Use Cases: Whether you're in healthcare, finance, or AI startups, Keylabs.ai supports diverse industries with customisable solutions.
The Process of Using Keylabs.ai for Labelling
Using Keylabs.ai for data labelling is a straightforward process. Here’s a step-by-step guide to help you get started:
- Sign Up for an Account: Visit Keylabs.ai and create your account.
- Upload Your Data: Easily upload your raw data, whether it's images, videos, text, or audio files, to the platform.
- Define Labelling Criteria: Specify the criteria for labelling to ensure that annotations meet your project’s needs.
- Utilize Automated Suggestions: Take advantage of the AI-driven automated labelling suggestions to speed up the process.
- Review and Edit Labels: Collaborate with team members to review and refine the labels for accuracy.
- Export Your Annotated Data: Once your data is labelled, export it in your desired format, ready for training machine learning models.
Best Practices for Effective Data Labelling
To ensure that your data labelling process is as effective as possible, consider the following best practices:
- Invest in Quality Training: Provide comprehensive training to your labellers or team members to ensure they understand how to apply labels accurately.
- Implement Regular Audits: Periodic checks on labelled data help maintain quality and consistency across datasets.
- Utilize Active Learning: Active learning allows the model to identify ambiguous cases, directing human annotators to focus on these instances for improved data quality.
- Maintain Clear Documentation: Keeping up-to-date documentation about the labelling process and guidelines helps ensure everyone is on the same page.
- Engage Feedback Loops: Create channels for feedback among your team and from automated tools to continuously improve your labelling practices.
The Future of Labelling Tools in Machine Learning
The future of labelling tools in machine learning is bright. As technology continues to evolve, we can expect even greater advancements in automation and user-friendliness within these tools. At Keylabs.ai, the integration of machine learning algorithms is revolutionizing the data annotation landscape, and as such tools become more sophisticated, they will further enhance the efficiency and accuracy of the labelling process.
In the coming years, we can anticipate:
- Enhanced Automation: Increased capabilities for automated labelling will streamline processes, reducing the need for extensive human involvement.
- Integration of Advanced AI: More sophisticated AI capabilities will likely provide even smarter suggestions and improve overall accuracy.
- User-centered Design: Labelling tools will continue to evolve toward more intuitive and user-friendly interfaces, making them accessible to novices.
- Focus on Ethics: The industry may see a growing emphasis on ethical labelling practices, ensuring fairness and transparency in AI systems.
Conclusion
In conclusion, the role of labelling tools in machine learning is indispensable. They not only facilitate the development of robust models but also enhance the overall quality of data analysis. As we look forward to the future, platforms like Keylabs.ai stand at the forefront of this transformation, offering innovative solutions and exceptional tools that can meet the diverse needs of businesses across various industries.
Investing in effective labelling tools is essential for any organization looking to thrive in the data-driven era of machine learning. Embrace the future, enhance your data labelling processes, and position your business for unparalleled success!