Harnessing the Power of Machine Learning Data Annotation for Business Growth
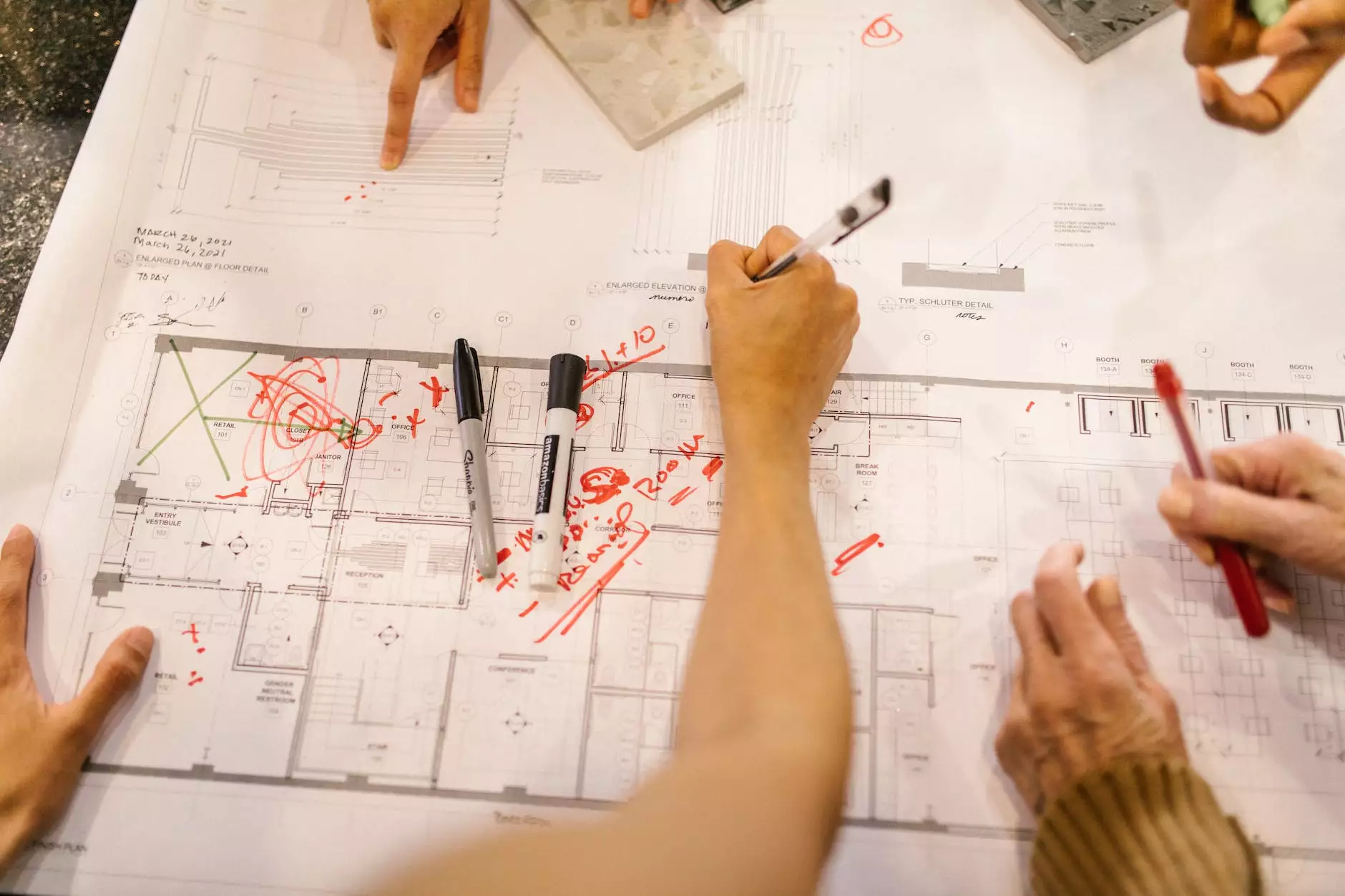
The landscape of business is constantly evolving, and one of the most transformative forces at play is machine learning data annotation. This innovative approach enhances not only technological systems but also fundamentally reshapes organizational strategies, processes, and outcomes. In this comprehensive article, we will delve deep into the significance of data annotation in machine learning, and how businesses across sectors such as Home Services, Keys & Locksmiths can leverage this technology to improve their operations and drive growth.
Understanding Machine Learning and Data Annotation
To grasp the importance of machine learning data annotation, it's vital to first understand the core concepts of machine learning itself. Machine learning involves the use of algorithms and statistical models that enable a system to perform tasks without accumulating instructions explicitly. In simpler terms, it allows machines to learn from data, identify patterns, and make decisions based on that data.
However, to train these machine learning models effectively, substantial amounts of labeled data are required. This is where data annotation comes into play. Data annotation is the process of labeling data, which can include images, text, audio, and video, to make it understandable for machine learning models. It provides the context that machines need to learn accurately, ensuring that they can perform tasks such as object recognition, sentiment analysis, and predictive analytics efficiently.
The Significance of Data Annotation in Business
The need for effective data annotation in today's business environment cannot be overstated. Here’s why:
- Improved Accuracy: Well-annotated data diminishes errors in machine learning predictions and classifications, leading to more accurate outcomes.
- Increased Efficiency: Automating certain processes can save time and resources, allowing businesses to focus on core competencies.
- Enhanced Customer Experience: By understanding customer behaviors and preferences through data analysis, businesses can personalize services and products, leading to higher satisfaction.
- Cost Reduction: Effective data annotation can streamline operations, reducing the operational costs of businesses, particularly in sectors like Home Services.
The Process of Data Annotation
To obtain high-quality machine learning models, a robust annotation process is paramount:
1. Data Collection
Begin by gathering raw data from diverse sources. This could be customer feedback, images, transactional data, etc.
2. Data Preprocessing
Next, clean the data to remove any inconsistencies or irrelevant information that could skew results.
3. Data Annotation
In this stage, data is tagged with appropriate labels to provide contextual meaning. For instance, images may be labeled to indicate the presence or absence of particular features.
4. Quality Assurance
One of the most critical stages involves checking the accuracy of annotations. High-quality labels are essential for training reliable models.
5. Model Training and Validation
Finally, the annotated data is used to train machine learning models, followed by rigorous testing to evaluate their performance.
Applications of Machine Learning Data Annotation in Home Services and Locksmiths
The Home Services and Keys & Locksmiths industries are ripe for innovation through machine learning data annotation. Here are some practical applications:
1. Intelligent Customer Support
By leveraging natural language processing (NLP), businesses can utilize machine learning models to analyze customer inquiries and provide automated yet personalized responses. This increases efficiency and enhances customer satisfaction.
2. Predictive Maintenance
For locksmith services, predictive models can analyze data from previous service requests to forecast when a customer might need maintenance or replacement services, leading to proactive outreach and improved service levels.
3. Customized Marketing Efforts
Using annotated customer data, businesses can create targeted marketing campaigns tailored to individual customer preferences and behaviors, greatly enhancing conversion rates.
4. Enhanced Security Features
By processing video feeds through machine learning algorithms trained with annotated data, locksmith services can develop advanced security systems that provide real-time alerts and insights.
Challenges in Data Annotation
Despite its immense potential, data annotation is not without challenges:
1. Time-Consuming Process
Annotating large datasets can be time-intensive, necessitating significant effort and resources.
2. Human Error
If done manually, there is always a risk of inconsistencies and errors in labeling, which can compromise the training of machine learning models.
3. Cost Implications
Outsourcing data annotation, while efficient, can incur significant costs, impacting the overall budget of smaller businesses.
Best Practices for Effective Data Annotation
To overcome the challenges of data annotation and optimize its benefits, businesses should consider the following best practices:
- Utilize Automation: Where possible, integrate tools and software that can automate parts of the annotation process to save time and reduce human error.
- Invest in Quality Control: Establish processes for regular reviews of the annotated data to ensure accuracy and consistency.
- Choose the Right Tools: Leverage sophisticated annotation tools that align with your specific requirements, whether it’s image recognition, text classification, or more complex forms.
- Train Your Team: Continuous training of staff involved in data annotation is crucial to maintain high quality and reliability.
The Future of Machine Learning Data Annotation
The evolution of technology indicates that the future of machine learning data annotation is promising. With advancements in artificial intelligence, it is expected that automation will further enhance the efficiency of the data annotation process. This progression will minimize costs and time, allowing businesses to focus on leveraging insights rather than data preparation.
Moreover, as the need for high-quality annotated data increases, there will be a growing demand for skilled professionals in this domain. The ability to interpret data accurately will become a critical skill set, further driving innovation in the industry.
Conclusion
In conclusion, machine learning data annotation represents a pivotal component for businesses interacting with vast amounts of data. From enhancing customer experiences to driving significant operational efficiencies, its applications transcend traditional boundaries, making it an invaluable asset across industries. For Home Services, Keys & Locksmiths, integrating effective data annotation practices is essential for staying competitive in an increasingly data-driven marketplace. Embracing these strategies today will pave the way for a smarter, more efficient tomorrow.
Investing in data annotation is not merely an option—it's a necessity for any forward-thinking business aiming to leverage the full potential of machine learning in today's dynamic environment.